Imputation Based Treatment Effect Estimators
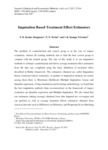
View/ Open
Date
2017-09-15Author
Dongmezo, P. B. Kenfac
Mwita, Peter N.
Tchwaket, I. R. Kamga
Metadata
Show full item recordAbstract
The problem of counterfactual and control group is at the core of impact
evaluation. Almost all existing methods aim to find the best control group to
compare with the treated group. The aim of this study is to use imputation
methods to estimate counterfactual and derive average treatment effect estimators
from the data sets completed using the basic definition of treatment effect
described in Rubin framework. The estimators obtained are called Imputation
Based Treatment Effects estimators. A number of imputation methods are tested,
among them there is Maximum likelihood, Multiple Imputation, Linear and
Quantile regressions. Using simulations and bootstrap methodology, we found that
the best imputation methods (data reconstruction) in the framework of impact
evaluation are Quantile regression and Multiple Imputation. We also found that
our estimators (taking average) obtained from data imputed are convergent and
can perform as well as average treatment effects estimators obtained from
classical methods such as Difference in Difference and Propensity Score Matching.Imputation Based Treatment Effect Estimators 24
Imputation Based Treatment Effect estimators are then tested on a program
(Lalonde data) and the results show that they can perform as well as existing
estimators and even better in certain cases especially when there is a shortage in
data.