24-Hours Load Forecasting Using a Hybrid of Genetic Algorithm (GA) and Particle Swarm Optimization (PSO) for Optimized Neural Network
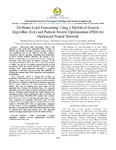
View/ Open
Date
2016Author
TabanInyasioSiriOgugu, Stephen
Abungu, N.O
Wekesa, Cyrus Wabuge
Metadata
Show full item recordAbstract
Short-term load forecasting (STLF) has emerged as one of the most important fields of study for efficient and reliable operation of power system. It plays a very significant role in the field of load flow analysis, contingency analysis, planning, scheduling and maintenance of power systems facilities, therefore, the system n costeffective is determine by accurate load forecast. Numerous researches have been done to improve accuracy of the conventional methods such as time series, regression analysis or ARMA and the use of artificial neural networks in load forecasting, ANN has shown lucrative results. But their training, with back-propagation algorithm or gradient algorithms is encounter long processing time, difficulty in selecting the optimal order of the components and trapping in local minima. These researches aimed at solving this problem by proposing a hybrid based on GA and PSO for train ANN and optimize the weights of ANN. The proposed method utilized the merit of GA ability for exploration of solution in space and PSO is widely well-known by social interchanges thinking ability. This help in reducing the search space for the algorithm thus reducing the iteration time. The proposed algorithm was tested using 24 hourly load data of different days i.e. Weekdays and weekends, the results obtained were compared to those results obtained by other researchers. It was observed that HGAPSO-ANN method has a better performance in terms of reducing and improving forecast error compared to Heuristic, PSO-ANN and GA-ANN methods. It was investigated that the method the lowest APE results is HGAPSO-ANN with an approximate minimum average error is 2.734% maximum average error 6.805%, therefore, a hybridized HGAPSO algorithm with ANN to help in reducing and improving forecast error. The suggested method was programmed under MATLAB 2016® software.