Distributive and Quantile Treatment Effects: Imputation Based Estimators Approach
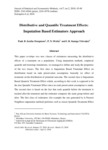
View/ Open
Date
2018Author
Dongmezo, Paul. B. Kenfac
Mwita, Peter. N.
Tchwaket, I. R. Kamga
Metadata
Show full item recordAbstract
This paper develops two new classes of estimators measuring the distributive
effects of a treatment on a population. Using imputation methods, empirical
quantile and bootstrap simulations, we managed to define and study the properties
of the two classes. The first class is Imputation Based Treatment Effect on
distribution based on rank preservation assumption, basically the effect of
treatment on the distribution of potential outcome. The second class is Imputation
Based Quantile Treatment Effect which, according to this work is supposed to be
the true Quantile Treatment Effect since no rank preservation assumption is made.
The second class is based on the fact that each quantile before the treatment is
tracked after the treatment and the estimator compares the same group before and
after. The first class of estimators (for example the one generated by k-Nearest
Neighbors imputation method) performs well as classic Quantile Treatment Effect
given the simulation result. When applied to Lalonde real data set, it performs
better than classic Quantile Treatment Effect and Firpo’s semi parametric
estimator especially for middle quantiles. Also, we found that there is a significant
difference between the two classes of estimators meaning that the bias caused by
rank preservation assumption is quite significant.