Performance of Imputation Methods towards Increasing Percentage of Missing Values
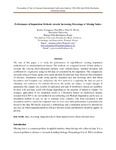
View/ Open
Date
2018-04Author
Brice, Kenfac D. P.
Mwita, Peter N.
Roger, Kamga T. I.
Metadata
Show full item recordAbstract
The aim of this paper is to study the performance of eightdifferent existing imputation
methodsused on simulatedand real dataset. The methods are compared in term of their ability to
estimate the missing observationsand estimate some statistics(mean, standard deviation and
coefficient of a regression) using the full data set completed by the imputation. The comparisons
are made using root mean square error, mean absolute deviationand bias observed after estimation
of statistics. Simulation results using specific simulated data and bootstrap show that Mean
Imputation and Complete case analysisare the best method in completing the data set and in
obtaining best estimators for statistics.However, the results are subject to major changes if
parameters like sample size, number of replication and type of distribution chosen are modified.
In short with real data, result will change depending on the structure of dataset to impute. For
example, application of the simulation results to a Rwandan dataset on smallholder farmers
revealed that k-NN is the best method in reconstructing and Multiple Imputation can be used as
imputation method in case we are to estimate some statistics. Our final conclusion is that
imputation methods cannot be compared since in most cases their performance is parametrically
linked to the data. We finally proposed a methodology and a simulation protocol to identify for
any data set which imputationmethod will give the best results and therefore should be applied in
priority.
Key words. Bias, Bootstrap, Imputation,Root Mean Squared Error, Mean Absolute error.